In the age of digitalization and globalization, the large amount of data is not only a central challenge but also a chance for industrial manufacturing and production. Decision-makers in all manufacturing industries are increasingly required to know how to interpret and use big data in production engineering. In this interview with Thomas Mair, we explore the relevance of artificial intelligence and its ongoing developments for production engineering, as well as the opportunities and risks associated with its implementation. We also delve into some of the most exciting use cases for manufacturing and its role in driving the fourth industrial revolution, also known as Industry 4.0.
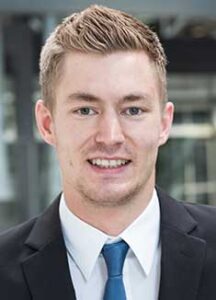
M. Sc. Thomas Mair from the Institute for Machine Tools and Industrial Management, TUM School of Engineering and Design, gives insights into the latest developments in Artificial Intelligence (AI) in production engineering.
Why is it important to deal with AI now?
Thomas Mair: So far, AI has only found its way into our everyday lives or into production technology in particular cases. But this situation changes rapidly. Recent developments, such as the chatbot ChatGPT, have impressively shown that AI is no longer in its infancy and has a major impact on society. The transfer of AI into production technology is also currently gaining momentum. One of our research projects, ProdBot, is testing a digital chatbot to provide the operational staff with process information in an industrial production environment (Research Project ProdBot). The two chatbots gain information from different sources. ChatGPT pulls the information from the internet, while ProdBot will be trained with production information.
To test the current maturity of the GPT technology, I asked ChatGPT, probably the best-known chatbot, to answer the following question. Then I checked the validity of the statements. This is a good example of what collaboration with AI in a production engineering company could look like in the future.
We asked ChatGPT: What are the opportunities and risks of AI in manufacturing?
ChatGPT: “AI offers a lot of opportunities in manufacturing, including increased efficiency, reduced costs, and improved product quality. However, it also comes with certain risks, including job displacement and ethical concerns.
– Reduced costs: AI can help to automate repetitive tasks, reduce labor costs and increase output without sacrificing quality.
– Improved product quality: AI can help to identify defects in products during production, leading to better quality control and fewer defective products.
– Predictive maintenance: AI can help to predict when equipment is likely to fail, allowing for proactive maintenance that can reduce downtime and save costs.
Risks:
– Job displacement: As AI is increasingly used in manufacturing, it may lead to job displacement as some tasks become automated.
– Ethical concerns: AI can be programmed to make decisions that may not align with ethical or moral values, which can lead to potential harm to employees or consumers.
– Data security: The use of AI in manufacturing involves the collection and storage of large amounts of data, which can be vulnerable to cyber-attacks if not properly secured.”
Thomas Mair: As you can see, ChatGPT is delivering valuable information and logical arguments at first sight. Nevertheless, you should not blindly trust it. The context of information is sometimes wrong and if one asks for the sources, then the content and the mentioned source frequently do not agree.
What are the most popular use cases of AI in production?
Thomas Mair: Popular use cases in production technology are Predictive Maintenance (PM) or Predictive Quality (PQ).
PM can be used to optimize the maintenance cycles of machines. The current condition of the machine or the failure of the machine is predicted on the basis of the machine data, e.g. the acceleration data of CNC machines. In this way, maintenance intervals can be adjusted and machine failure can be prevented. The use of AI results in lower maintenance costs and safer production.
In PQ, for example, the quality of products is assessed on the basis of images. After the analysis, a decision is made on whether the product meets the specified quality requirements. In many cases, the analysis results can be used for a statement about the cause of defects and to initiate appropriate measures.
Another application that is currently being researched intensively is the operation of Reinforcement Learning for planning tasks. For example, job scheduling or CNC program planning can be taken over.
What role does AI in manufacturing play in Industry 4.0?
Thomas Mair: Industry 4.0 involves the intelligent networking of machines and processes in the industry with the help of information and communication technologies. The resulting data volumes can easily overwhelm humans or current methods of data analysis. And this is precisely where AI can bring its advantages to bear. If the models run on GPUs, processes and analyses can be parallelized for acceleration. In addition, the large amount and the breadth of data offer the potential to make the models’ predictions more precise and robust.
How does the new certificate program “AI in Production Engineering” help bridge the knowledge gap in regards to AI in manufacturing industries?
Thomas Mair: In the new certificate program AI in Production Engineering, we will teach a basic understanding of AI, especially Machine Learning (ML). This is done along the methodology Knowledge Discovery in Databases (KDD), which contains the essential steps for the successful development of an ML method. During the certificate program, the entire process chain is run through, from the preparation of the data to the evaluation of the prediction accuracy, in order to make AI comprehensible for the users. The focus of the KDD process is on modeling. Different AI approaches (e.g. grey-box and black-box methods) are considered and their advantages and disadvantages are highlighted. The program was tested before within internal trainings at the institute: The combination of theoretical basics and practical implementation in Python has been very well received by the participants so far.
After finishing the program, the participants understand the theoretical basis of ML techniques and are able to apply some of them. In addition, they are capable of identifying the key challenges of ML techniques.